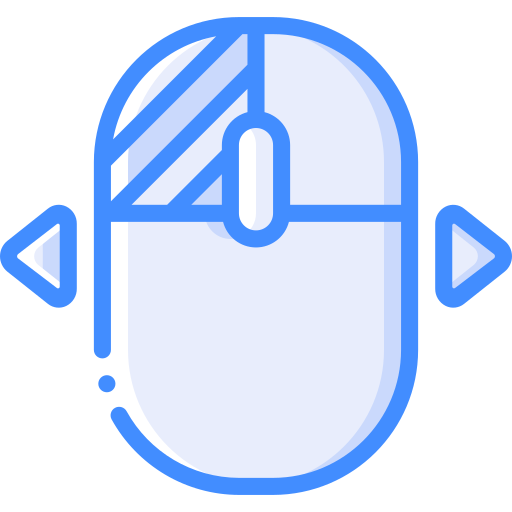
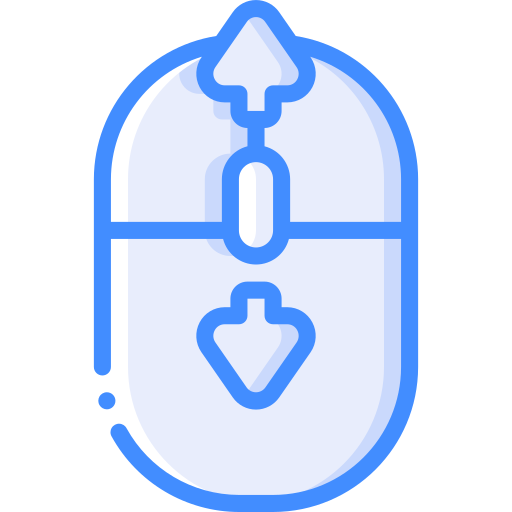
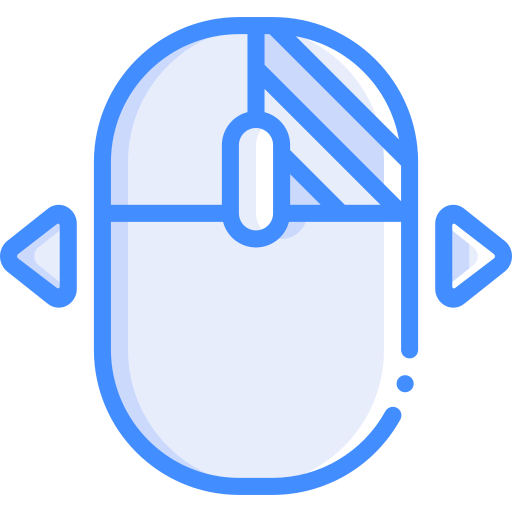
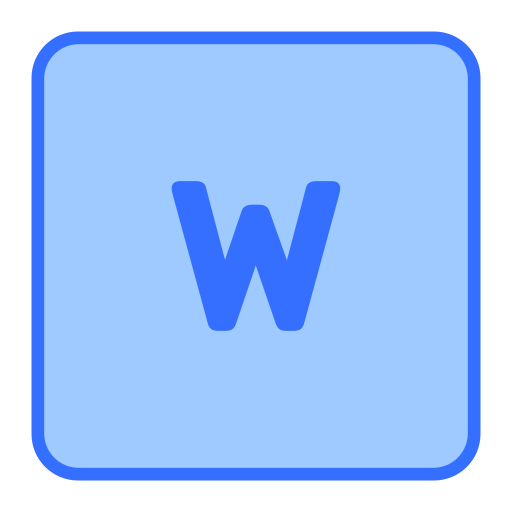
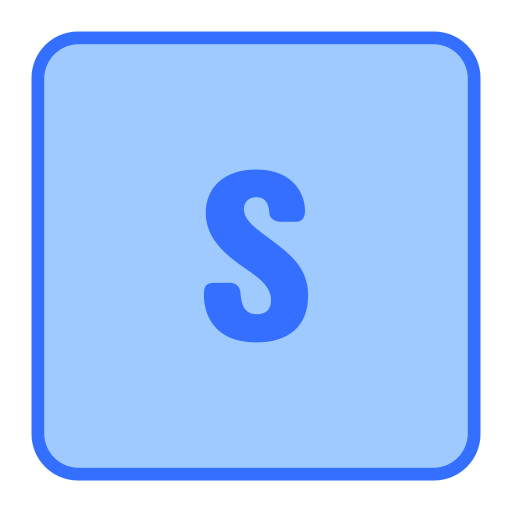
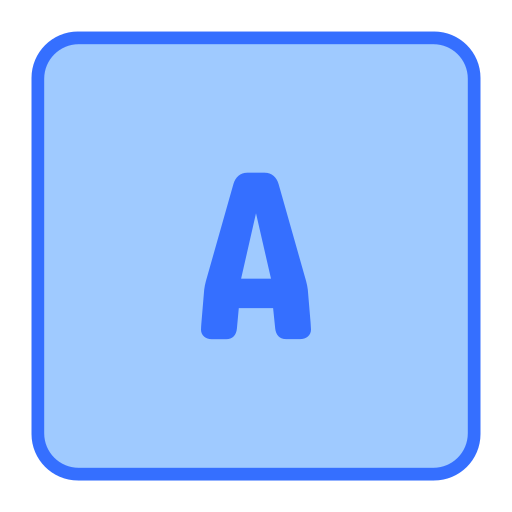
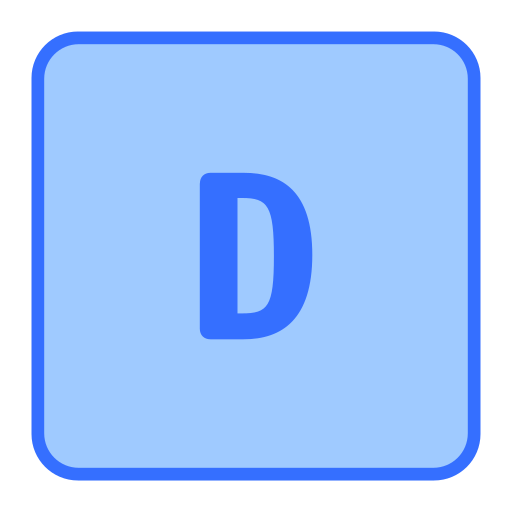
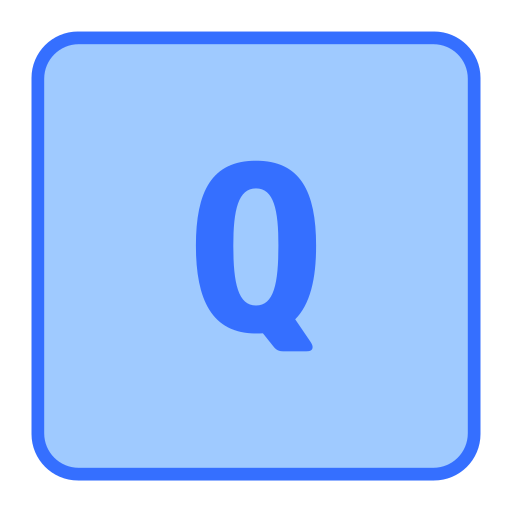
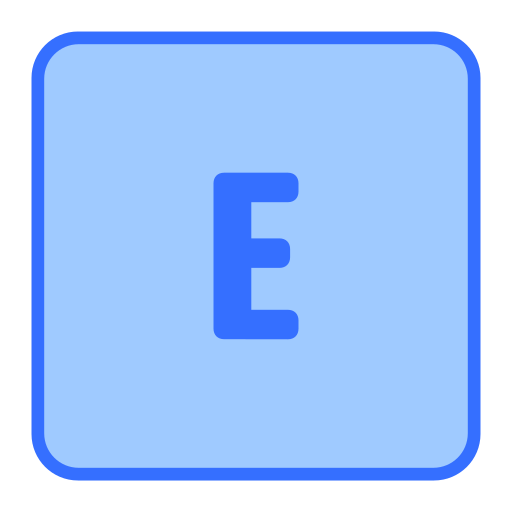
Note: Results are downsampled 4 times for efficient online rendering. Also, we do not mask out any points for fair comparison.
We introduce Geo4D, a method to repurpose video diffusion models for monocular 3D reconstruction of dynamic scenes. By leveraging the strong dynamic prior captured by such video models, Geo4D can be trained using only synthetic data while generalizing well to real data in a zero-shot manner. Geo4D predicts several complementary geometric modalities, namely point, depth, and ray maps. It uses a new multi-modal alignment algorithm to align and fuse these modalities, as well as multiple sliding windows, at inference time, thus obtaining robust and accurate 4D reconstruction of long videos. Extensive experiments across multiple benchmarks show that Geo4D significantly surpasses state-of-the-art video depth estimation methods, including recent methods such as MonST3R, which are also designed to handle dynamic scenes.
Overview of Geo4D. During training, video conditions are injected by locally concatenating the latent feature of the video with diffused geometric features \( \mathbf{z}_t^{\mathbf{X}}, \mathbf{z}_t^{\mathbf{D}}, \mathbf{z}_t^{\mathbf{r}} \) and are injected globally via cross-attention in the denoising U-Net, after CLIP encoding and a query transformer. During inference, iteratively denoised latent features \( \hat{\mathbf{z}}_0^{\mathbf{X}}, \hat{\mathbf{z}}_0^{\mathbf{D}}, \hat{\mathbf{z}}_0^{\mathbf{r}} \) are decoded by the fine-tuned VAE decoder, followed by multi-modal alignment optimization for coherent 4D reconstruction.
Note: Results are downsampled 4 times for efficient online rendering. Also, we do not mask out any points for fair comparison.
Attribute to our group-wise inference manner and prior geometry knowledge from pretrained video diffusion model, our model successfully produces consistent 4D geometry under fast motion. For more comparisons, please visit the comparison page.
Our method generalizes to various scenes with different 4D objects and performs robustly against different camera and object motion. For more results, please visit the result page.
Our method achieves state-of-the-art performance in video depth estimation and produces temporally consistent, highly detailed depth maps for diverse in-the-wild sequences.
Zeren Jiang was supported by Clarendon Scholarship. This work were also supported by ERC s-UNION and EPSRC EP/Z001811/1 SYN3D.
We thank Junyi Zhang for discussing the experiments of MonST3R with us.
We also thank Stanislaw Szymanowicz, Ruining Li, Runjia Li, Jianyuan Wang, Minghao Chen, Jinghao Zhou, Gabrijel Boduljak and Xingyi Yang for helpful suggestions and discussions.
@misc{jiang2025geo4d,
title={Geo4D: Leveraging Video Generators for Geometric 4D Scene Reconstruction},
author={Zeren Jiang and Chuanxia Zheng and Iro Laina and Diane Larlus and Andrea Vedaldi},
year={2025},
eprint={2504.07961},
archivePrefix={arXiv},
primaryClass={cs.CV},
url={https://arxiv.org/abs/2504.07961},
}